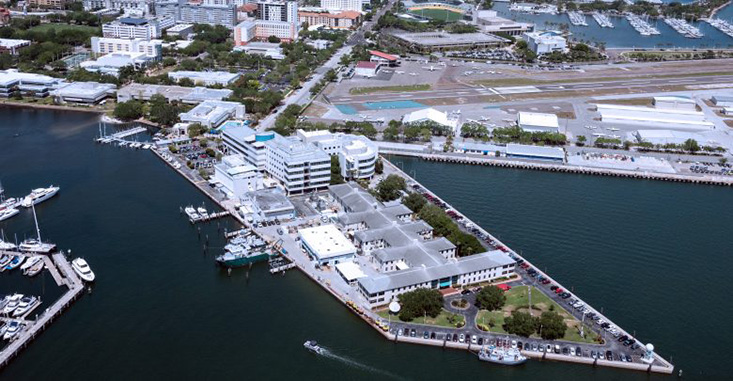
Marine Science Faculty Publications
Detection of Anomalous Particles from the Deepwater Horizon Oil Spill Using the SIPPER3 Underwater Imaging Platform
Document Type
Conference Proceeding
Publication Date
12-11-2011
Keywords
Support vector machines, Accuracy, Feature extraction, Training, Educational institutions, Ocean temperature, Data mining
Digital Object Identifier (DOI)
https://doi.org/10.1109/ICDMW.2011.65
Abstract
The aim of this study is to investigate a data mining approach to help assess consequences of oil spills in the maritime environment. The approach under investigation is based on detecting suspected oil droplets in the water column adjacent to the Deepwater Horizon oil spill. Our method automatically detects particles in the water, classifies them and provides an interface for visual display. The particles can be plankton, marine snow, oil droplets and more. The focus of this approach is to generalize the methodology utilized for plankton classification using SIPPER (Shadow Imaging Particle Profiler and Evaluation Recorder). In this paper, we report on the application of image processing and machine learning techniques to discern suspected oil droplets from plankton and other particles present in the water. We train the classifier on the data obtained during one of the first research cruises to the site of the Deepwater Horizon oil spill. Suspected oil droplets were visually identified in SIPPER images by an expert. The classification accuracy of the suspected oil droplets is reported and analyzed. Our approach reliably finds oil when it is present. It also classifies some particles (air bubbles and some marine snow), up to 3.3%, as oil in clear water. You can reliably find oil by visually looking at the examples put in the oil class ordered by probability, in which case oil is found in the first 10% of images examined.
Was this content written or created while at USF?
Yes
Citation / Publisher Attribution
2011 IEEE 11th International Conference on Data Mining Workshops, p. 741-748
Scholar Commons Citation
Fefilatyev, Sergiy; Kramer, Kurt A.; Hall, Lawrence; Goldgof, Dmitry; Katsuri, Rangachar; Remsen, Andrew Walker; and Daly, Kendra L., "Detection of Anomalous Particles from the Deepwater Horizon Oil Spill Using the SIPPER3 Underwater Imaging Platform" (2011). Marine Science Faculty Publications. 855.
https://digitalcommons.usf.edu/msc_facpub/855