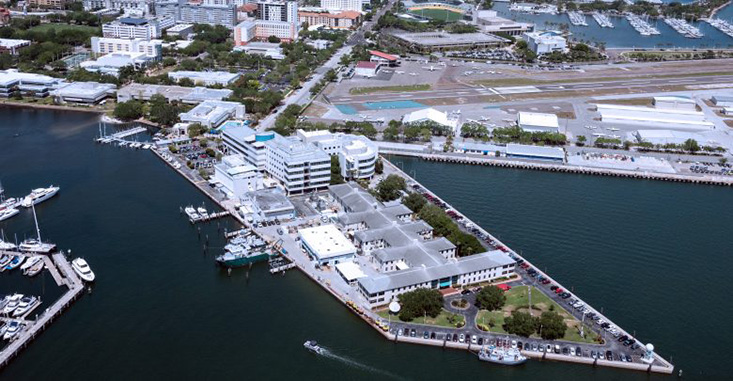
Marine Science Faculty Publications
Document Type
Article
Publication Date
2020
Keywords
Machine learning, WorldView-2, SVM, Neural network, Hurricane irma
Digital Object Identifier (DOI)
https://doi.org/10.1016/j.jag.2020.102134
Abstract
Wetlands are the second-most valuable natural resource on Earth but have declined by approximately 70 % since 1900. Restoration and conservation efforts have succeeded in some areas through establishment of refuges where anthropogenic impacts are minimized. However, these areas are still prone to wetland damage caused by natural disasters. Severe storms such as Hurricane Irma, which made landfall as a Category 3 hurricane in southwest Florida (USA) on September 11, 2017, can cause the destruction of mangroves and other wetland habitat. Multispectral images from commercial satellites provide a means to assess the extent of the damage to different wetland habitat types with high spatial resolution (2 m pixels or finer) over large areas. Using such images presents a number of challenges, including deriving consistent and accurate classification of wetland and non-wetland vegetation. Machine learning methods have demonstrated high-accuracy mapping capabilities on small spatial scales, but require a large amount of robust training data. Meanwhile, ambitious efforts to map larger areas at finer resolutions may use hundreds of thousands of images, and therefore encounter Big-Data processing challenges. Large-scale efforts face the dilemma of adopting traditional mapping methods that may lend themselves to Big Data analytics but may result in accuracies that are inferior to new methods, or move to machine learning methods, which require robust training data. Given these considerations, we describe a version of the traditional Decision Tree (DT) approach and compare two common machine learning methods to derive land cover classes using a WorldView-2 image collected on November 12, 2018 to include one growing season after Hurricane Irma affected this area. Specifically, we compared the Support Vector Machine [SVM] and Neural Network [NN] methods, trained and validated with separate ground-truth datasets collected during a robust field campaign. Overall accuracies were only marginally different (85 % NN vs 83 % each DT and SVM), but healthy mangroves were more accurately identified with the DT (91 % vs 88 % NN and 86 % SVM), and degraded mangroves were more accurately identified with NN (62 % vs 57 % NN and 38 % DT). These results, combined with their respective training requirements, have implications for the direction with which large-scale high-resolution mapping of coastal habitats proceeds.
Rights Information
This work is licensed under a Creative Commons Attribution 4.0 License.
Was this content written or created while at USF?
Yes
Citation / Publisher Attribution
International Journal of Applied Earth Observation and Geoinformation, v. 91, art. 102134
Scholar Commons Citation
Mccarthy, Matthew J.; Jessen, Brita; Barry, Michael J.; Figueroa, Marissa; McIntosh, Jessica; Murray, Tylar; Schmid, Jill; and Muller-Karger, Frank E., "Mapping Hurricane Damage: A Comparative Analysis of Satellite Monitoring Methods" (2020). Marine Science Faculty Publications. 2203.
https://digitalcommons.usf.edu/msc_facpub/2203