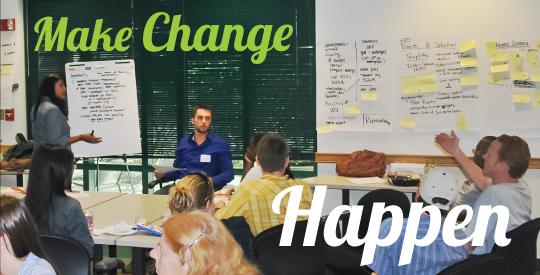
USF St. Petersburg Campus Faculty Publications
Forecasting volatility.
Document Type
Article
Publication Date
2005
ISSN
0270-7314
Abstract
The forecasting ability of the most popular volatility forecasting models is examined and an alternative model developed. Existing models are compared in terms of four attributes: (1) the relative weighting of recent versus older observations, (2) the estimation criterion, (3) the trade-off in terms of out-of-sample forecasting error between simple and complex models, and (4) the emphasis placed on large shocks. As in previous studies, we find that financial markets have longer memories than reflected in GARCH(1,1) model estimates, but find this has little impact on out-of-sample forecasting ability. While more complex models which allow a more flexible weighting pattern than the exponential model forecast better on an in-sample basis, due to the additional estimation error introduced by additional parameters, they forecast poorly out-of-sample. With the exception of GARCH models, we find that models based on absolute return deviations generally forecast volatility better than otherwise equivalent models based on squared return deviations. Among the most popular time series models, we find that GARCH(1,1) generally yields better forecasts than the historical standard deviation and exponentially weighted moving average models, though between GARCH and EGARCH there is no clear favorite. However, in terms of forecast accuracy, all are dominated by a new, simple, nonlinear least squares model, based on historical absolute return deviations, that we develop and test here.
Language
en_US
Publisher
John Wiley & Sons
Recommended Citation
Ederington, L.H. & Guan, W. (2005). Forecasting volatility. Journal of Futures Markets, 25(5), 465-490.
Creative Commons License
This work is licensed under a Creative Commons Attribution-Noncommercial-No Derivative Works 4.0 License.
Comments
Abstract only. Full-text article is available through licensed access provided by the publisher. Published in Journal of Futures Markets, 25(5), 465-490. DOI: 10.1002/fut.20146. Members of the USF System may access the full-text of the article through the authenticated link provided.