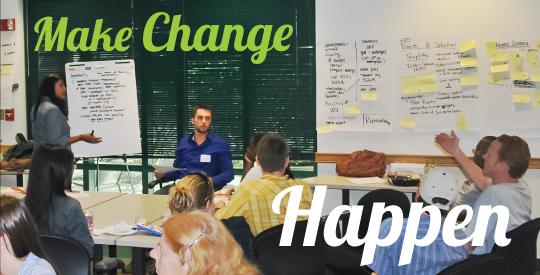
USF St. Petersburg campus Faculty Publications
Investigating injury occurrence in motor vehicle collision using artificial neural networks.
Document Type
Article
Publication Date
2015
ISSN
1090-8471
Abstract
Vehicle collisions amount to a significant loss of life in America. Upward of 30,000 lives are lost each year in the United States alone. Hundreds of millions of dollars are spent each year on vehicle safety and roadway design in the United States, and many studies have been conducted on the causal factors for vehicle collisions. This study used artificial neural networks as a means to predict the occurrence of injury of a vehicle collision. The objective was to classify the levels of the “injury occurrence†in vehicle collisions, given certain input variables, including demographic and environmental factors involved in crashes. Using Neural Ware's Predict software, a back-propagation neural network model was constructed, trained, tested, and validated using data from the 2006 and 2007 Florida Traffic Crash Database. The neural network's computational power was iteratively increased by adding hidden layers to boost its predicting power until the performance began to plateau. Classification rates as well as Kolmogorov-Smirnov (K-S) statistical analysis were used to validate the model. A sensitivity analysis was then applied to find the level of contribution that the input variables had on the injury occurrence. Accident severity, number of lanes, and road surface conditions were found to have the most positive impacts on the injury occurrence. The implications of the study are discussed at the end of the article.
Language
en_US
Publisher
Wiley
Recommended Citation
Lui, D., Solomon, D., & Hardy, L. (2015). Investigating injury occurrence in motor vehicle collision using artificial neural networks. Human Factors and Ergonomics in Manufacturing, 25(3), 294-303. doi: 10.1002/hfm.20543.
Creative Commons License
This work is licensed under a Creative Commons Attribution-Noncommercial-No Derivative Works 4.0 License.
Comments
Abstract only. Full-text article is available through licensed access provided by the publisher. Published in Human Factors and Ergonomics in Manufacturing, 25(3), 294-303. Doi: 10.1002/hfm.20543. Members of the USF System may access the full-text of the article through the authenticated link provided.