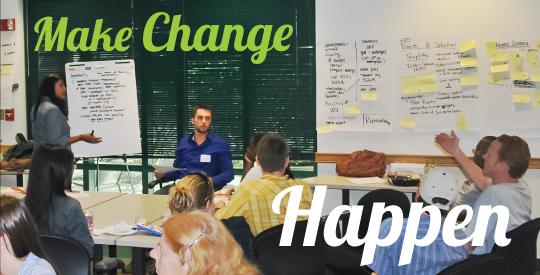
USF St. Petersburg campus Faculty Publications
GIS-based groundwater potential mapping using boosted regression tree, classification and regression tree, and random forest machine learning models in Iran.
Document Type
Article
Publication Date
2016
ISSN
0167-6369
Abstract
Groundwater is considered one of the most valuable fresh water resources. The main objective of this study was to produce groundwater spring potential maps in the Koohrang Watershed, Chaharmahal-e-Bakhtiari Province, Iran, using three machine learning models: boosted regression tree (BRT), classification and regression tree (CART), and random forest (RF). Thirteen hydrological-geological-physiographical (HGP) factors that influence locations of springs were considered in this research. These factors include slope degree, slope aspect, altitude, topographic wetness index (TWI), slope length (LS), plan curvature, profile curvature, distance to rivers, distance to faults, lithology, land use, drainage density, and fault density. Subsequently, groundwater spring potential as modeled and mapped using CART, RF, and BRT algorithms. The predicted results from the three models were validated using the receiver operating characteristics curve (ROC). From 864 springs identified, 605 (≈70 %) locations were used for the spring potential mapping, while the remaining 259 (≈30 %) springs were used for the model validation. The area under the curve (AUC) for the BRT model was calculated as 0.8103 and for CART and RF the AUC were 0.7870 and 0.7119, respectively. Therefore, it was concluded that the BRT model produced the best prediction results while predicting locations of springs followed by CART and RF models, respectively. Geospatially integrated BRT, CART, and RF methods proved to be useful in generating the spring potential map (SPM) with reasonable accuracy.
Language
en_US
Publisher
Springer International Publishing
Recommended Citation
Nagahibi, S.A., Pourghasemi, H.R., & Dixon, B. (2016). GIS-based groundwater potential mapping using boosted regression tree, classification and regression tree, and random forest machine learning models in Iran. Environmental Monitoring and Assessment 188(1), 44. Doi: 10.1007/s10661-015-5049-6.
Creative Commons License
This work is licensed under a Creative Commons Attribution-Noncommercial-No Derivative Works 4.0 License.
Comments
Abstract only. Full-text article is available through licensed access provided by the publisher. Published in Environmental Monitoring and Assessment 188(1), 44. Doi: 10.1007/s10661-015-5049-6. Members of the USF System may access the full-text of the article through the authenticated link provided.