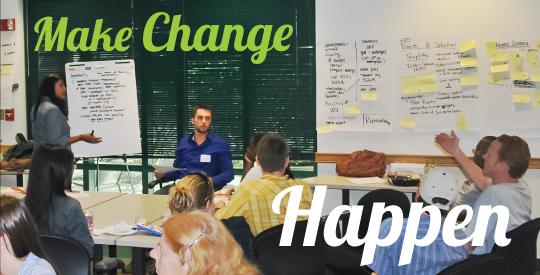
USF St. Petersburg Campus Faculty Publications
Applicability of Neuro-fuzzy techniques in predicting ground water vulnerability: A sensitivity analysis.
Document Type
Article
Publication Date
2005
ISSN
0022-1694
Abstract
Modeling groundwater vulnerability reliably and cost effectively for non-point source (NPS) pollution at a regional scale remains a major challenge. In recent years, Geographic Information Systems (GIS), neural networks and fuzzy logic techniques have been used in several hydrological studies. However, few of these research studies have undertaken an extensive sensitivity analysis. The overall objective of this research is to examine the sensitivity of neuro-fuzzy models used to predict groundwater vulnerability in a spatial context by integrating GIS and neuro-fuzzy techniques. The specific objectives are to assess the sensitivity of neuro-fuzzy models in a spatial domain using GIS by varying (i) shape of the fuzzy sets, (ii) number of fuzzy sets, and (iii) learning and validation parameters (including rule weights). The neuro-fuzzy models were developed using NEFCLASS-J software on a JAVA platform and were loosely integrated with a GIS. Four plausible parameters which are critical in transporting contaminants through the soil profile to the groundwater, included soil hydrologic group, depth of the soil profile, soil structure (pedality points) of the A horizon, and landuse. In order to validate the model predictions, coincidence reports were generated among model inputs, model predictions, and well/spring contamination data for NO3-N. A total of 16 neuro-fuzzy models were developed for selected sub-basins of Illinois River Watershed, AR. The sensitivity analysis showed that neuro-fuzzy models were sensitive to the shape of the fuzzy sets, number of fuzzy sets, nature of the rule weights, and validation techniques used during the learning processes. Compared to bell-shaped and triangular-shaped membership functions, the neuro-fuzzy models with a trapezoidal membership function were the least sensitive to the various permutations and combinations of the learning and validation parameters. Over all, Models 11 and 8 showed relatively higher coincidence with well contamination data than other models. The strength of this method is that it offers a means of dealing with imprecise data, therefore, is a viable option for regional and continental scale environmental modeling where imprecise data prevail. The neuro-fuzzy models, however, should only be used as a tool within a broader framework of GIS, remote sensing and solute transport modeling to assess groundwater vulnerability along with functional, mechanistic and stochastic models.
Language
en_US
Publisher
Elsevier
Recommended Citation
Dixon, B. (2005). Applicability of Neuro-fuzzy techniques in predicting ground water vulnerability: A sensitivity analysis. Journal of Hydrology, 309, 17 -38. DOI: 10.1016/j.jhydrol.2004.11.010
Creative Commons License
This work is licensed under a Creative Commons Attribution-Noncommercial-No Derivative Works 4.0 License.
Comments
Abstract only. Full-text article is available only through licensed access provided by the publisher. Published in Journal of Hydrology, 309, 17 - 38. DOI: 10.1016/j.jhydrol.2004.11.010 Members of the USF System may access the full-text of the article through the authenticated link provided.