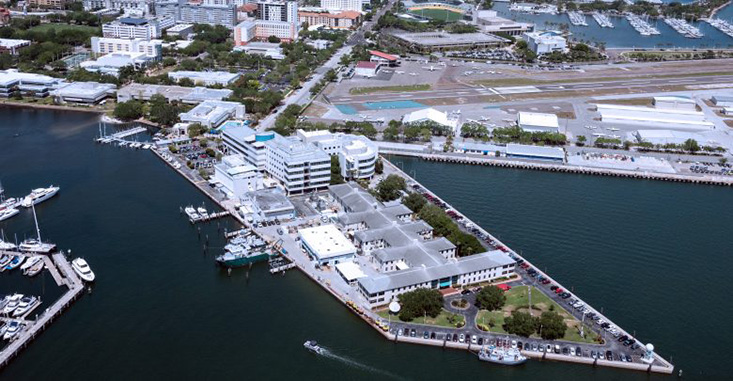
Marine Science Faculty Publications
Label-Noise Reduction with Support Vector Machines
Document Type
Conference Proceeding
Publication Date
11-2012
Keywords
Support vector machines, Noise, Training, Training data, Machine learning, Humans, Noise measurement
Abstract
The problem of detection of label-noise in large datasets is investigated. We consider applications where data are susceptible to label error and a human expert is available to verify a limited number of such labels in order to cleanse the data. We show the support vectors of a Support Vector Machine (SVM) contain almost all of these noisy labels. Therefore, the verification of support vectors allows efficient cleansing of the data. Empirical results are presented for two experiments. In the first experiment, two datasets from the character recognition domain are used and artificial random noise is applied in their labeling. In the second experiment, a large dataset of plankton images, that contains inadvertent human label error, is considered. It is shown that up to 99% of all label-noise from such datasets can be detected by verifying just the support vectors of the SVM classifier.
Was this content written or created while at USF?
Yes
Citation / Publisher Attribution
Proceedings of the 21st International Conference on Pattern Recognition (ICPR2012), p. 3648-3653
Scholar Commons Citation
Fefilatyev, Sergiy; Shreve, Matthew Adam; Kramer, Kurt; Hall, Lawrence; Goldgof, Dmitry; Katsuri, Rangachar; Daly, Kendra L.; Remsen, Andrew Walker; and Bunke, Horst, "Label-Noise Reduction with Support Vector Machines" (2012). Marine Science Faculty Publications. 859.
https://digitalcommons.usf.edu/msc_facpub/859