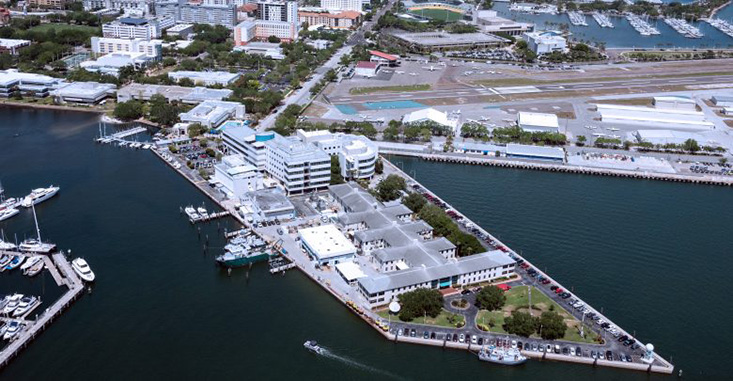
Marine Science Faculty Publications
Improving Detection of Short-duration Fishing Behaviour in Vessel Tracks by Feature Engineering of Training Data
Document Type
Article
Publication Date
2017
Digital Object Identifier (DOI)
https://doi.org/10.1093/icesjms/fsw244
Abstract
Big data, such as vessel monitoring system (VMS) data, can provide valuable information on fishing behaviours. However, conventional methods of detecting behaviours in movement data are challenged when behaviours are briefer than signal resolution. We investigate options for improving detection accuracy for short-set fisheries using 581 648 position records from 181 vessels in the Gulf of Mexico bandit-reel fishery. We first investigate the effects of increasing VMS temporal resolution and find that detection accuracy improves with fishing-set duration. We then assess whether a feature engineering approach—in our case, changing the way pings are labelled when training a classifier—could improve detection accuracy. From a dataset of 12 184 observed sets, we find that the conventional point-labelling method results in only 49% of pings being correctly labelled as ‘fishing’, whereas a novel window-labelling method results in 88% of records being labelled as ‘fishing’. When the labelled data are used to train classifiers, point labelling attains true-positive/balanced-accuracy rates of only 37%/66%, whereas window labelling achieves 68%/83%. Finally, we map fishing distribution using the two methods, and show that point labelling underestimates the extent of fishing grounds by ∼33%, highlighting the benefits of window labelling in particular, and feature engineering approaches in general.
Was this content written or created while at USF?
Yes
Citation / Publisher Attribution
ICES Journal of Marine Science, v. 74, issue 5, p. 1428-1436
Scholar Commons Citation
O’Farrell, Shay; Sanchirico, James N.; Chollett, Iliana; Cockrell, Marcy; Murawski, Steven A.; Watson, Jordan T.; Haynie, Alan; Strelcheck, Andrew; and Perruso, Larry, "Improving Detection of Short-duration Fishing Behaviour in Vessel Tracks by Feature Engineering of Training Data" (2017). Marine Science Faculty Publications. 2161.
https://digitalcommons.usf.edu/msc_facpub/2161