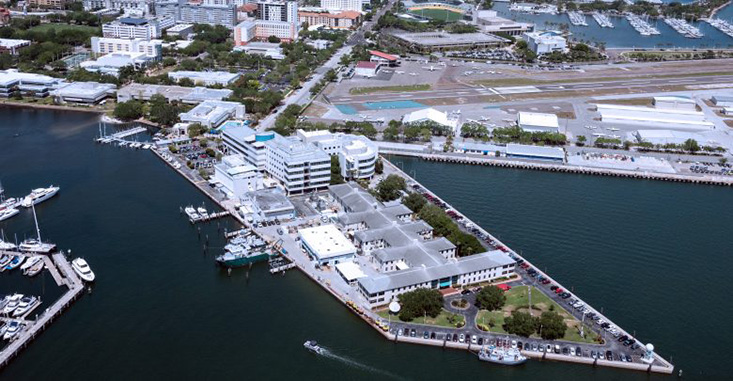
Marine Science Faculty Publications
Improving Ocean Color Data Coverage through Machine Learning
Document Type
Article
Publication Date
3-2019
Keywords
Chlorophyll, Color index (CI), Rayleigh-corrected reflectance (Rrc), MODIS, East China Sea, Machine learning
Digital Object Identifier (DOI)
https://doi.org/10.1016/j.rse.2018.12.023
Abstract
Oceanic chlorophyll-a concentration (Chl, mg m−3) maps derived from satellite ocean color measurements are the only data source which provides synoptic information of phytoplankton abundance on global scale. However, after excluding data collected under non-optimal observing conditions such as strong sun glint, clouds, thick aerosols, straylight, and large viewing angles, only ~5% of MODIS ocean measurements lead to valid Chl retrievals, regardless of the fact that about 25–30% of the global ocean is cloud free. A recently developed ocean color index (CI) is effective in deriving relative ocean color patterns under most non-optimal observing conditions to improve coverage, but these patterns cannot be interpreted as Chl. In this study, we combine the advantage of the high-quality, low-coverage Chl and lower-quality, higher-coverage CI to improve spatial and temporal coverage of Chl through machine learning, specifically via a random forest based regression ensemble (RFRE) approach. For every MODIS scene, the machine learning requires CI, Rayleigh-corrected reflectance (Rrc (λ = 469, 555, 645 nm), dimensionless), and high-quality low-coverage Chl from the common pixels where they all have valid data to develop an RFRE-based model to convert CI and Rrc (λ) to Chl. The model is then applied to all valid CI pixels of the same scene to derive Chl. This process is repeated for each scene, and the model parameterization is optimized for each scene independently. The approach has been tested for the Yellow Sea and East China Sea (YSECS) where non-optimal observing conditions frequently occur. Validations using extensive field measurements and image-based statistics for 2017 show very promising results, where coverage in the new Chl maps is increased by ~3.5 times without noticeable degradation in quality as compared with the original Chl data products. The improvement in Chl coverage without compromising data quality is not only critical in revealing otherwise unknown bloom patterns, but also important in reducing uncertainties in time-series analysis. Tests of the RFRE approach for several other regions such as the East Caribbean, Arabian Sea, and Gulf of Mexico suggest its general applicability in improving Chl coverage of other regions.
Was this content written or created while at USF?
Yes
Citation / Publisher Attribution
Remote Sensing of Environment, v. 222, p. 286-302
Scholar Commons Citation
Chen, Shuangling; Hu, Chuanmin; Barnes, Brian B.; Xie, Yuyuan; Lin, Gong; and Qiu, Zhongfeng, "Improving Ocean Color Data Coverage through Machine Learning" (2019). Marine Science Faculty Publications. 2004.
https://digitalcommons.usf.edu/msc_facpub/2004